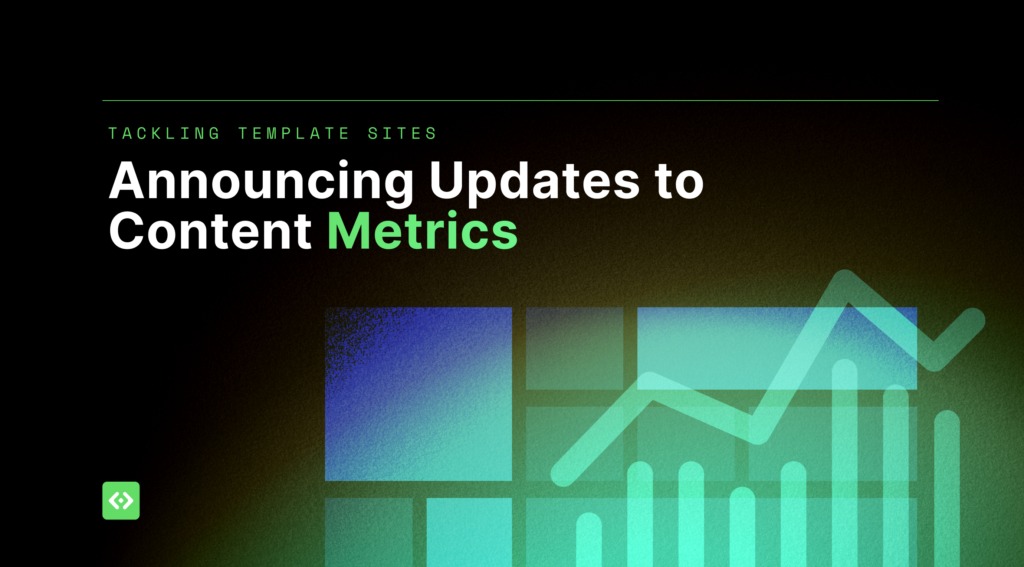
It’s been a little over a month since we released our new research portal, and the reception has been both encouraging, and educational! We’ve brought in clients across all our usage tiers, and they come from all parts of the supply chain. Through conversations with those clients, we’re constantly honing in on where the product provides the most value, and rapidly iterating based on that feedback.
Our prospects often initially consider DeepSee a buy-side solution, so it’s been great to receive a lot of positive feedback from SSPs, supply-curators, and ad-managers. Turns out, it’s helpful to have partners speaking the same language when talking about quality & compliance, and the research portal reports & list export metrics are helping our clients do just that.
This batch of features largely support businesses wanting to keep a watchful eye on templated sites, which are characterized by generic content, and non-unique design (adtech historians will recall that these are 2/5 of ANA/4As MFA definition). These sites are largely in the long-tail, and mostly affect buyers who don’t use target lists. Since they’re easy (and only getting easier) to make, it’s a moderation problem across any platform offering self-serve access to programmatic ad spend.
What’s New:
Design Uniqueness Measurement:
What is it about template sites that make them look so…same-y? This is something we’re starting to capture with hard data by measuring the prevalence with which we encounter certain site design choices. Template sites are largely in the long-tail, and tend to favor a handful of site-building tools for their ease-of-use, such as WordPress (#1 by a mile), and Squarespace. Our 1st pass at a measure of design uniqueness is simple, but effective:
- For WordPress sites, design choices are captured in terms of which plugins & themes are in use.
- For Squarespace sites, design choices are captured in terms of the plugins & templates detected.
For each component type (plugins, themes, and templates) we look for that exact combination of components in use across all the sites we visit. For example, take daysinncollinsville[.]com:
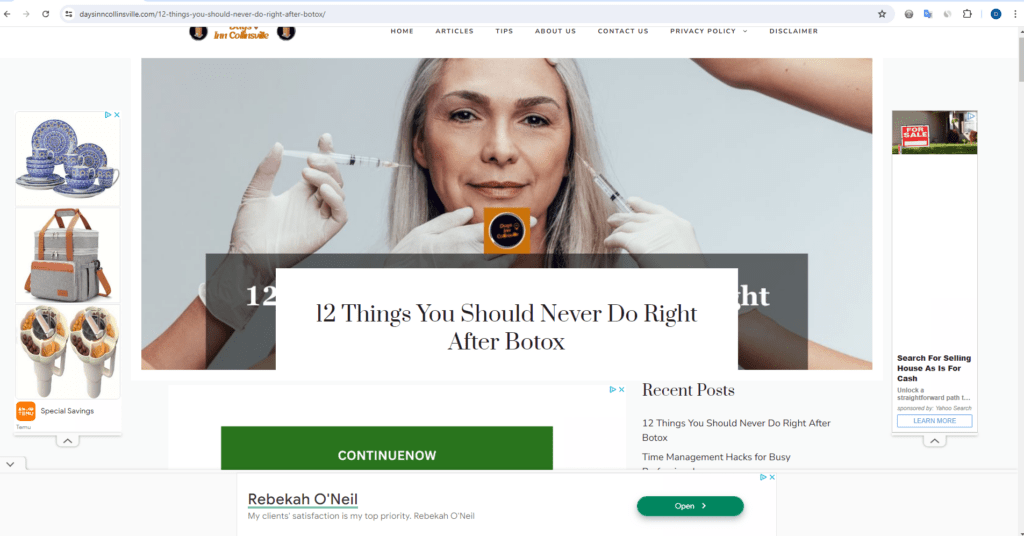
Without going too much in the weeds: this is an old SEO hacking trick where someone buys an expired domain from a small business that has many existing links, and turns it into a clickbait-belching template site. How did this come to our attention when looking at design uniqueness metrics?
- This site was built with WordPress, and used only one plugin: “gp-premium”
- We found nearly 2000 other root domains using ONLY that plugin, making it quite an outlier in terms of non-unique plugin profile (most sites have a fairly distinct combination of plugins)
- The “generatepress” theme they chose is extremely common, being seen across nearly 40,000 other root domains.
Author Trust Signals:
How often have you been on a low-quality site and rolled your eyes upon seeing the “admin” author byline?
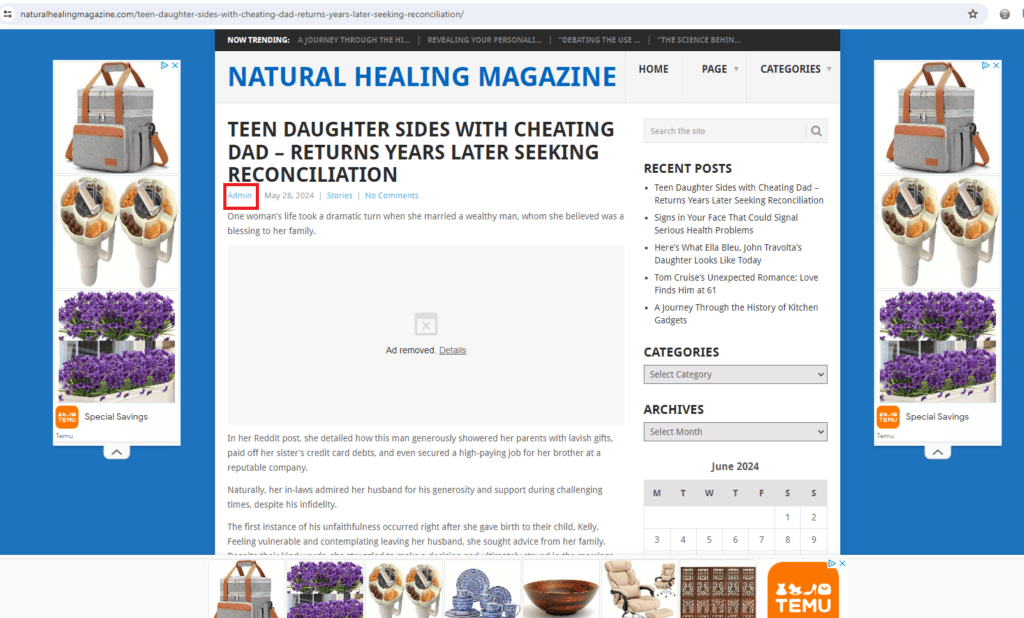
Well, now that’s captured in a handy metric, along with another obvious low-quality author signal; we found that template sites often will insert the domain name (minus TLD) as author. As we grow our database of authors, the sky’s the limit for what can be inferred; right now, we’re looking for something that could resemble an actual human name (something lazy template crankers can’t be bothered to fake, apparently).
Identifying Low Quality Text and Spammy Content:
Many have asked for AI content detection, and the fact is: it’s simply not something that can be done reliably, by any entity, at this point in time. However, that’s not to say that we came up empty in our search for metrics! We were inspired by Bahri et. al. & their 2020 whitepaper (Generative Models are Unsupervised Predictors of Page Quality: A Colossal-Scale Study) which demonstrated the potential for detecting spam & low quality text using classifiers meant to find machine-generated text. To quote their findings: “detectors trained to discriminate human vs. machine-written text are effective predictors of web- pages’ language quality, outperforming a baseline supervised spam classifier.”
They identified 4 distinct types of documents which are scored the worst by models like this, and those are:
- Machine translated text
- Essay farms
- Attempts at Search Engine Optimization
- NSFW content
When evaluated against our database of web content, we were able to reproduce the results, and are happy to present our users now with some measure of content that appears spammy, or low-quality!
Feedback from our Users:
“Mediavine’s core business strategy has always been to monetize and support human content creators. We’ve turned to DeepSee to aid us in monitoring quality across our publisher set of over 11,000 sites, giving advertisers the reassurance necessary to successfully capitalize on the open internet. What started as an effort to truly understand how the buy-side evaluated our inventory, quickly developed into a partnership to develop signals such as design uniqueness, author trust, and content quality.”
– Brad Hagmann, Senior Vice President – Advertising Technology at Mediavine
“DeepSee tools enable our teams to get a […] head start on troubleshooting partner issues. This saves our team valuable time allowing us to scale and spend more time focused on driving revenue with more partners.
-Scott Wolke, Director, Publisher Operations at The Arena Group
Coming Up Next:
Soon our clients will have access to app store data for iOS and Android!
About deepsee.io
deepsee.io is dedicated to improving the digital advertising ecosystem through providing detailed publisher risk and quality data. By focusing on innovation and client needs, we’re helping advertisers navigate the complexities of digital marketing with greater confidence and effectiveness.
For more details on our new portal, including our 7-day free trial for new users, book a demo here.